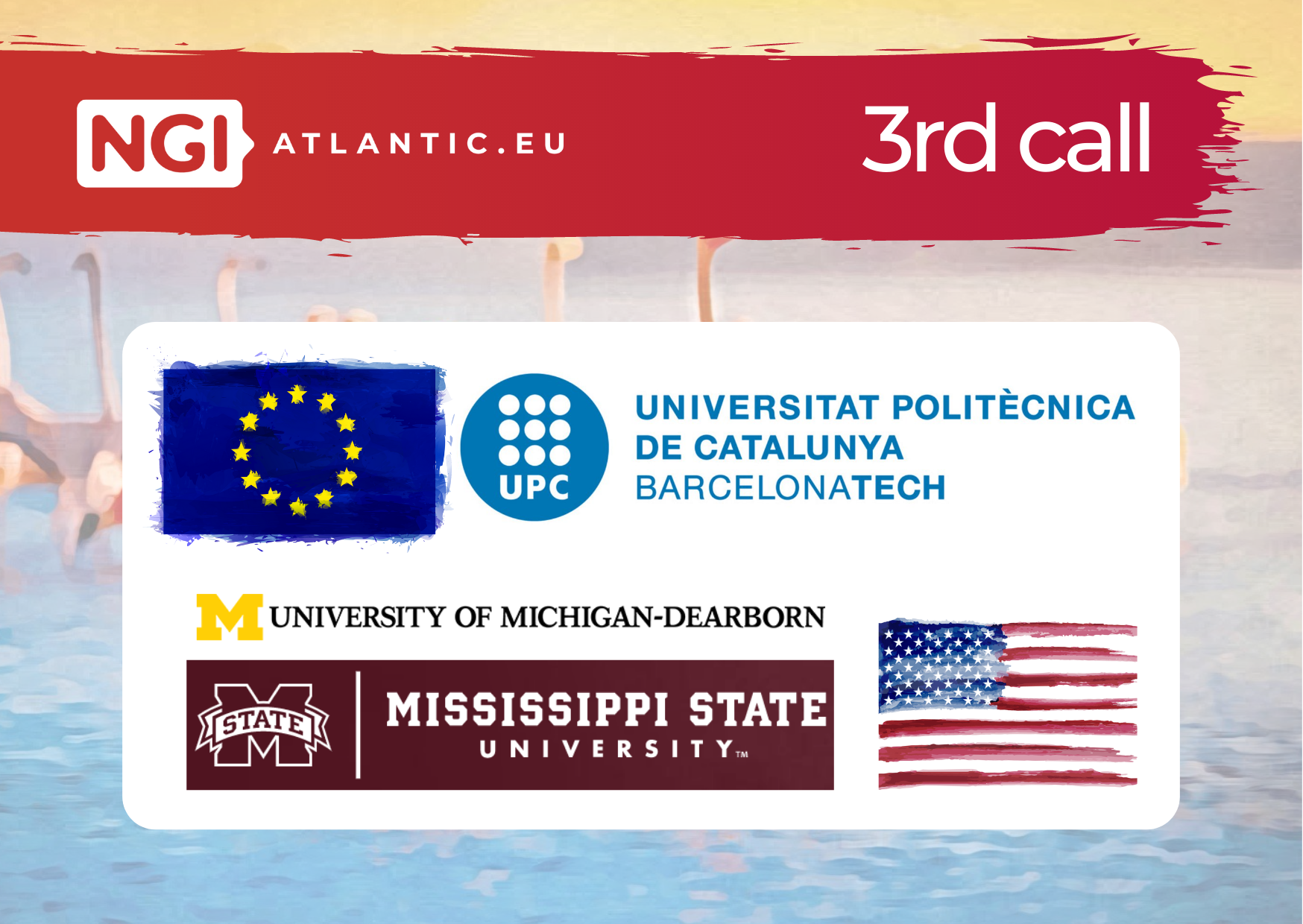
Project Coordinator (EU) :
UNIVERSITAT POLITECNICA DE CATALUNYACountry of the EU Coordinator :
SpainOrganisation Type :
AcademiaProject participants :
US: Texas Tech University (TTU)
Lu Wei is an assistant professor at the Department of Computer Science at Texas Tech University (TTU). He obtained a Ph.D. degree from Aalto University, Finland, in 2013. He held postdoctoral positions at University of Helsinki, Finland, from 2013 to 2015 and at Harvard University, USA, from 2015 to 2016.
US: Mississippi State University (MSU):
Chun-Hung Liu is an assistant professor at the Department of Electrical and Computer Engineering of Mississippi State University (MSU). He obtained a Ph.D.degree from University of Texas at Austin, USA, in 2011.
EU:
Felix Freitag is an associate Professor at the Department of Computer Architecture of UPC. He obtained a PhD degree from the UPC in 1998. He coordinated the FP7 CLOMMUNITY project (2013-2015). His recent research focuses on community clouds and federated learning. Felix supervised several PhD theses. The full list of his publications is available at https://futur.upc.edu/FelixUrsErichFreitag.
State of US partner :
MississippiTexas
Starting date :
Adaptive decentralized federated learning in wireless mesh network (FLESHNET)
Experiment description
The increase of the computing capacity of end user-owned devices and the appearance of lightweight machine learning frameworks have led to the situation that machine learning is nowadays available at user devices, while just a few years ago machine learning was exclusive to cloud providers. Federated learning has recently appeared as a promising machine learning approach which respects the privacy of the data. We envision novel federated learning applications in which any node can participate in the provision of data and in the training of machine learning models. For this to happen, federated learning building blocks must be extended and evaluated. These building blocks will enable the autonomy of the participants for taking self-determined decisions, facilitate the ownership of machine learning models and data, and enable decentralized governance of the data. This scenario will be realistic in the near future as edge nodes of a federated learning network will receive an ever increasing amount of data coming from more and more nearby sensors.
Implementation plan :
The FLESHNET project will apply an experimentally driven research in which the development of federated learning designs will obtain feedback from the prototype experimentation, experimentally evaluated in the our testbed. The FLESHNET project is organized in three work packages (WPs) with two focus areas: WP1 (Design) and WP2 (Integration) is dedicated to design and integrate federated learning components in a deployable prototype. WP3 (Experimentation) conducts the experimentation in the wireless mesh network testbed. FLESHNET will have two iterations with short design and experiment cycles of three months. Initial experimental results at M3 will feed the research of the second cycle and to obtain final outcomes at M6.
During M1, the activities in WP1 will prepare the initial building blocks. From M2 to M4 the components will be integrated in a deployable prototype by WP2 and experimentation will be carried out by WP3. The first cycle finishes in M4 where WP1 carries out the analysis and feeds the results to WP2, to develop in M5 the improved design, and for being experimented in M6 by WP3 closing the loop.
The consolidation of the findings of WP1 in the second cycle will provide the results for objective 1, namely experimentally-driven design and integration of adaptive decentralized federated learning components. The consolidation of the findings of WP3 in the second cycle will provide the results for objective 2, namely experimental validation of federated learning in wireless mesh networks.
The experiments validate the designs in a relevant environment (i.e., an operational wireless mesh network with real nodes). Such an environment can classify as TRL 5.
Impacts :
Impact 1: Enhanced EU – US cooperation in Next Generation Internet, including policy cooperation.
Fleshnet has established a new successful collaboration of EU-US partners from the project team coming from three universities (TTU (US). MSU (US), UPC (EU)). With our progress and collaboration we could also raise the attention of two other European research groups (from AUEB and SEEU university), which triggered a collaboration on this topic. This collaboration resulted in a joint paper, which otherwise may not have happened.
Impact 2: Reinforced collaboration and increased synergies between the Next Generation Internet and the Tomorrow's Internet programmes.
The program of the U.S. partners is CNS (Computer and Network Systems) under the CISE (Computer and Information Science and Engineering) program. The impact the project of this NSF one includes: 1) verify the practical usefulness of the research findings on wireless sensor deployment of the U.S. partners; 2) verify whether the proposed repulsive processes are able to accurately model the performance of practical wireless networks; 3) assess the robustness and sensitivity of the proposed models for a given error tolerance. Synergies: 1) the research results of the U.S. partner will lead to key insights into the design and deployment of real-world ultra-dense networks for practitioners; 2) to evaluate the project findings, the EU-US collaboration provided the opportunity to work with Dr. Freitag’s team at UPC for experimental validation using FL based implementations; 3) the collaboration delivered building blocks for adaptive decentralized networks experimentally validated in the realistic conditions in the wireless mesh network testbed.
Impact 3: Developing interoperable solutions and joint demonstrators, contributions to standards.
The code for the experimentation has been made available in public gitlab repository and is thus shared for allowing others to build on our work. A demonstration paper about the testbed and experimentation was written by the project team. It was presented at CCNC 2022 hold as a virtual event.
Impact 4: An EU - US ecosystem of top researchers, hi-tech start-ups / SMEs and Internet-related communities collaborating on the evolution of the Internet.
We took advantage of scientific conferences which took place within the project duration and achieved three publications. The conferences were related to scientific communities which research on different aspects of the evolution of the Internet. Specifically, we published and presented the work done in the Fleshnet project in:
1. ACM/SIGCHI International Conference on the Internet of Things (IoT 2021)
2. IEEE 19th Annual Consumer Communications & Networking Conference (CCNC 2022)
3. International Conference on Information Technology & Systems (ICITS 2022)
Results :
In FLESHNET we studied the federated learning process in an heterogeneous environment in order to identify more adaptive designs for exploiting this heterogeneity. Federated learning uses a distributed computing infrastructure, therefore heterogeneity can be expected in several forms:
1) The quantity and quality of local data at each node may vary. For instance, local data may be acquired by sensors at or nearby the nodes, but the local circumstances of each node lead to a different number of training samples.
2) The computing capacity of the nodes can be different either by the proper hardware of the nodes or by concurrent executions of other applications at the node which reduce the available computing resources dedicated to the federated learning process.
3) For doing the exchange of the trained model some nodes may face limited network capacities, either due to permanent or dynamic network conditions.
Future Plan :
The practical experimentation of federated learning in a testbed deployed in a wireless city mesh network revealed resource usage patterns which gave evidence for the heterogeneity that exists in real edge computing infrastructure. It was shown how computing capacity of the nodes and bandwidth availability affect the federated learning process.
A more adaptive and decentralized design was experimented for the server and clients. Building blocks were designed which provided server-side adaptability and client-side decentralized decisions. The workflow for the interactions between clients and server was developed. The communication interfaces of the client and server were designed.
Applying the proposed design allows federated learning components to take into account different criteria for adapting or taking local decisions, such as the network situation, the computing capability, data privacy and the data sharing policy of each node.
The obtained results are not limited to the specific infrastructure and experimental environment. As machine learning moves into ever smaller computing devices with energy and computing limitations, applying the gained insights about adaptability and decentralization may be critical for being able to deliver the maximum outcome under important resource constraints. In this respect, the the Fleshnet partners have decided to exploit the momentum of the joint work and extend their collaboration with a new project proposal targeting the connected dynamic intelligence at the tiny edge.